Listen to this podcast on Spotify, Apple Podcasts, Podbean, Podtail, ListenNotes, TuneIn, PodChaser
Season 1, Episode 14
Host: Deepesh Patel, Editor, Trade Finance Global
Featuring: Michael Boguslavsky, Head of AI, Tradeteq
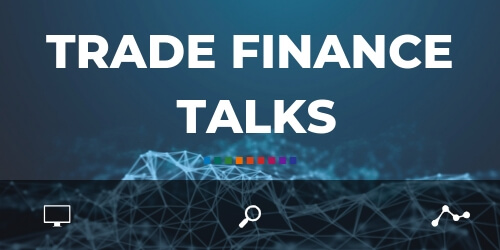
An interview at the Telegraph’s Future of Trade & Export Conference with Tradeteq’s Head of AI, on digitising trade finance with AI, machine learning, robotics and natural language processing
Deepesh Patel: I’m Deepesh Patel, Editor at Trade Finance Global.
Machine learning, artificial intelligence, robotics, natural language processing. It seems as though there are a number of disruptive opportunities to digitise trade finance. But I want to get into the nuts and bolts of these buzzwords and assess the real, viable applications of these technologies within international trade and finance.
The International Data Corporation predicts that worldwide spending on cognitive and AI systems will reach $77.6 billion USD by 2022. It is clear that AI has much to offer within the trade and receivables finance area.
Deepesh Patel: Today we’re at the Telegraph’s Future of Trade & Export conference, and I have the pleasure of being joined by Michael Boguslavsky, Head of AI at Tradeteq. Hi Michael, thank you for joining us on Trade Finance Talks.
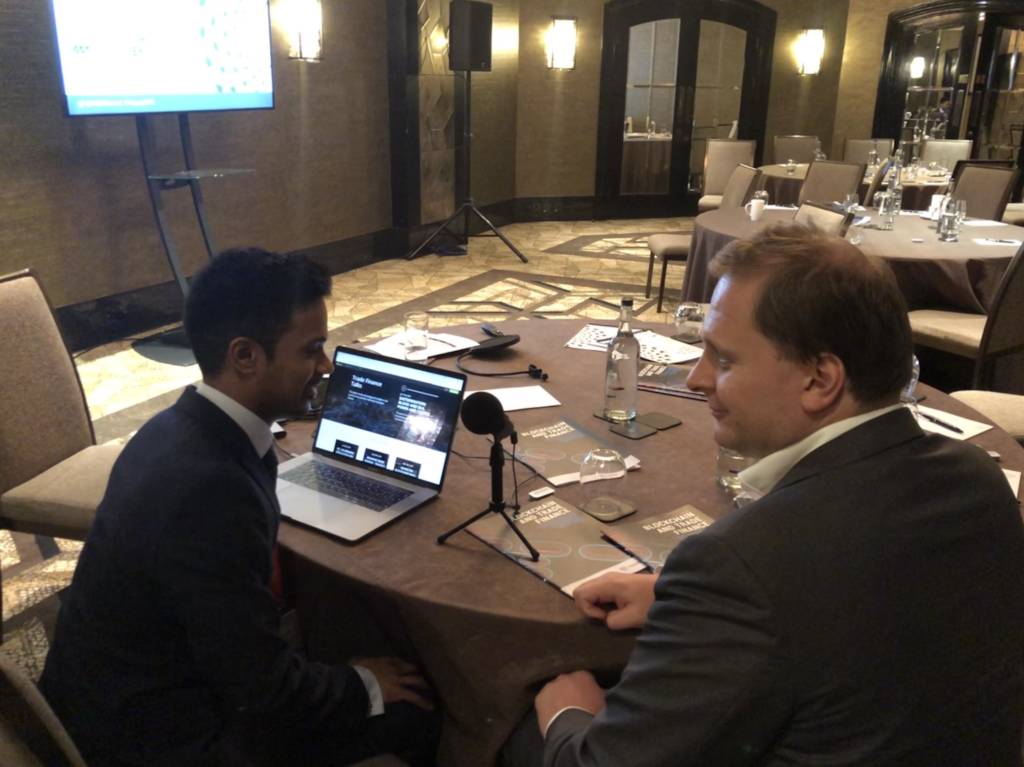
Host: Deepesh Patel, Editor, TFG (left)
Featuring: Michael Boguslavsky, Head of AI, Tradeteq (right)
Location: Juremiah Tower, London, Telegraph Future of Trade & Export Conference
So, in no more than 30 seconds, tell us your elevator pitch: who are you and what do you do at Tradeteq?
Michael Boguslavsky: At Tradeteq, we are creating a market for trade finance assets. Currently, the trade finance receivables space includes payables finance and letters of credit. This market remains the exclusive domain of banks. Unlike most other financial markets. If you’re an investor you can access many assets, commodities, mortgages and private loans, but it’s really difficult to access trade finance. What we are doing is creating a market network and infrastructure for investors to access trade finance assets.
DP: We often hear news about the US $1.5 trillion trade finance gap. What role does Tradeteq play within the trade finance distribution initiative that we reported on recently?
MB: Tradeteq provides the technology behind the Trade Finance Distribution (TFD) Initiative. This technology enables banks and non-bank originators to distribute their trade finance assets to other investors. These days, the banks can originate much more to finance than they are currently originating – there is a demand for it from their corporate clients. However, the regulations and capital rules for many countries – and for many banks – don’t allow them to scale up these exposures and the natural solution would be to distribute these exposures to investors. But, large institutional investors are not really equipped for decision making on trade finance assets. They don’t have systems for that and they don’t have models for that. That’s where we help by providing the technology for this origination and distribution.
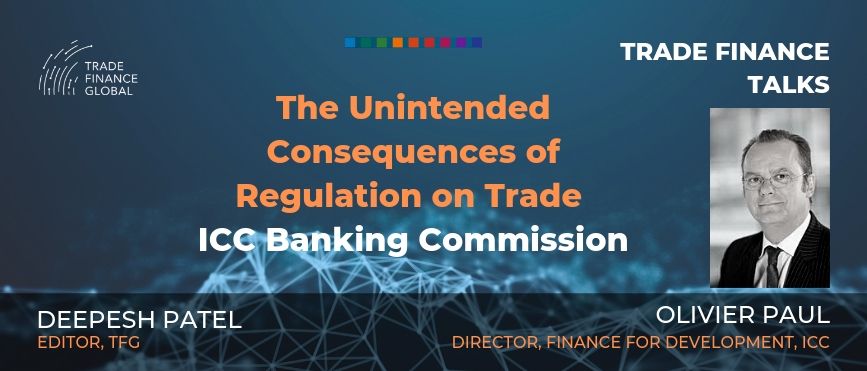
DP: OK, let’s move on to artificial intelligence. Is it just a buzzword?
MB: Well, it is it is a buzzword for sure – and a very trendy one! However, AI is useful when one needs to make a decision, based on large volumes of data. When you have repetitive transactions (which require analysis), and when it’s hard for a human to grasp this volume of data, then trade finance is perfectly suited to AI, with a large number of small repetitive transactions. These happen on a huge series of graphs; the supply chain graph, and grasping the interconnectedness of this graph is not that easy without the proper tools – and that’s what AI helps to do. We use AI to predict the timeliness and the extent of payment on trade finance receivables.
DP: Thank you. So, today we’re at the Telegraph’s Trade & Export Finance Conference. What were the key takeaways and also what was the vibe from the audience that you’ve been speaking to?
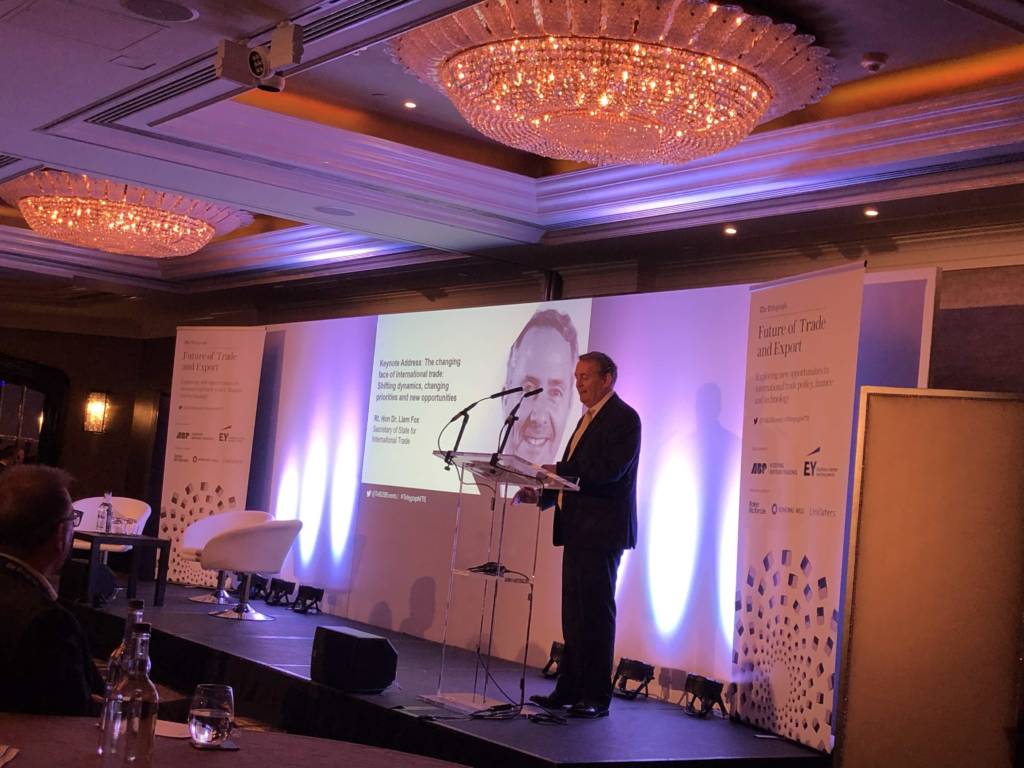
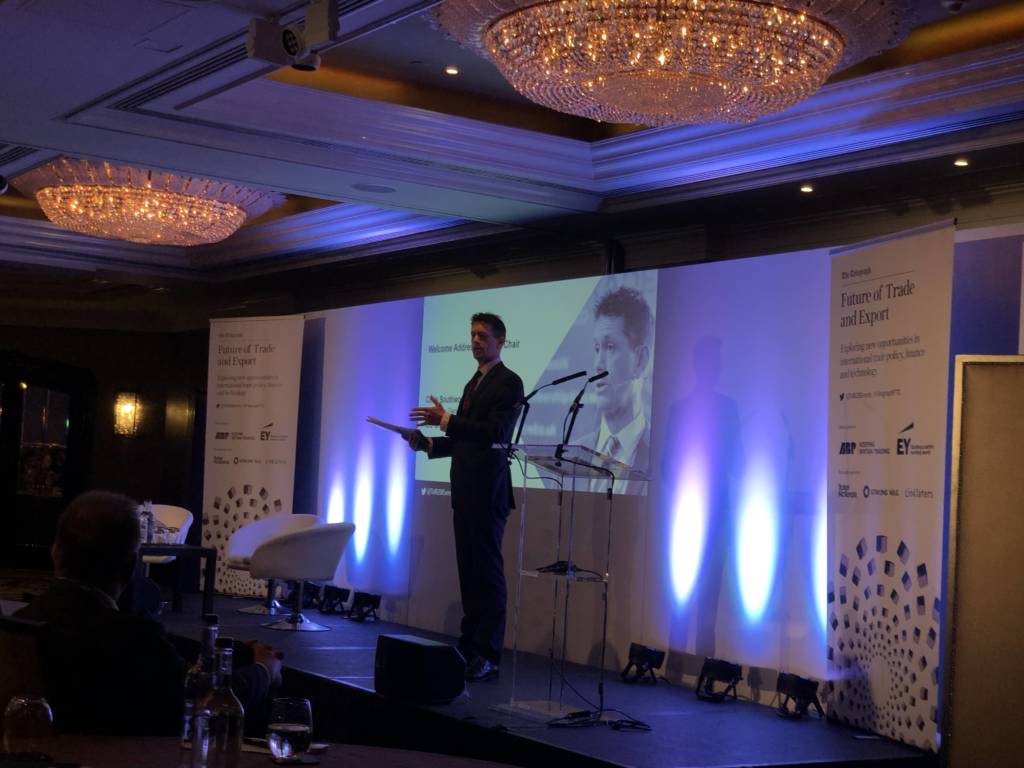
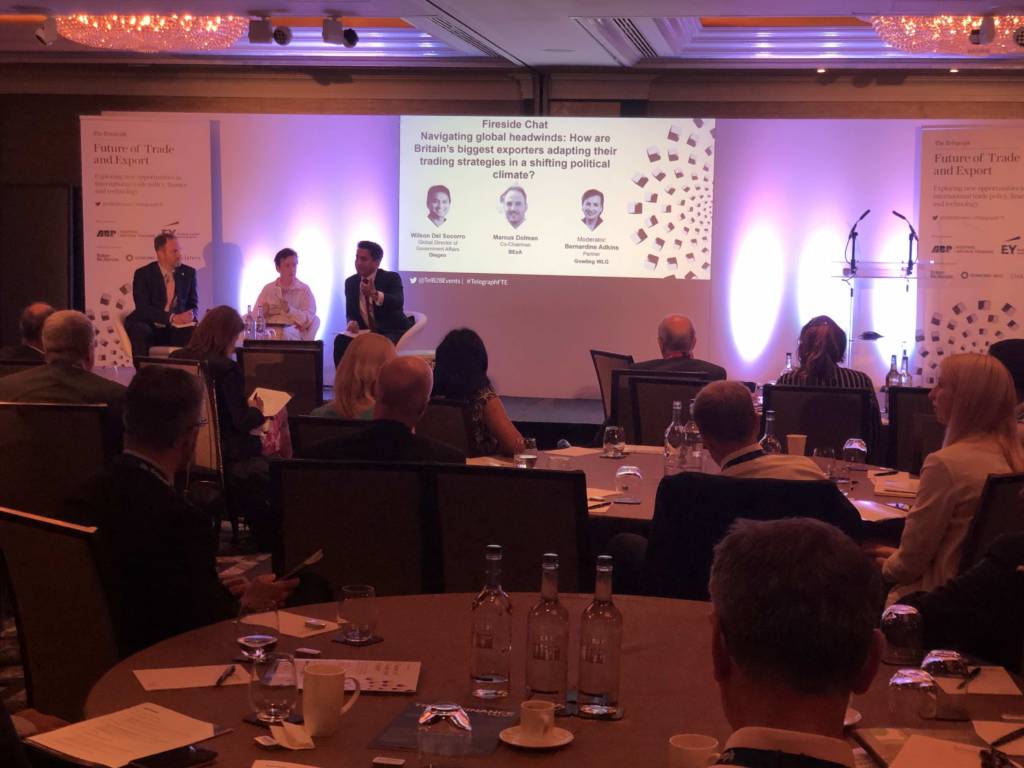
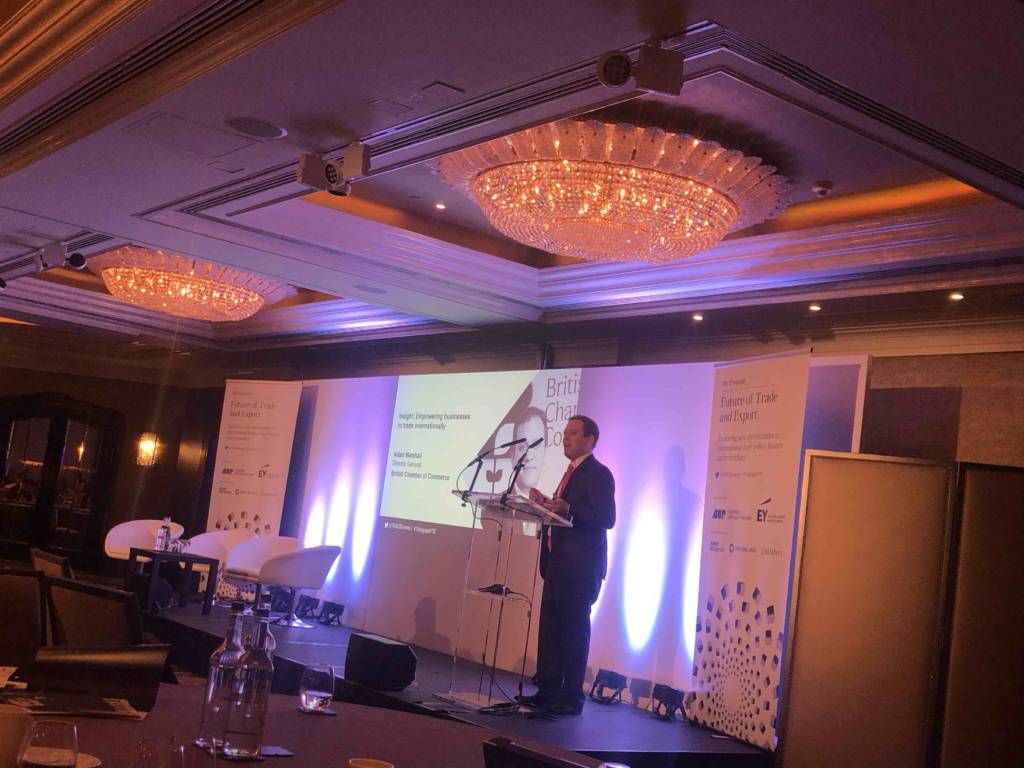
MB: Many people were interested in how AI could help them, and finding out who is actually using AI today. Adoption of AI is at relatively early stages. I would say it is more advanced in some economies, for example in Asia. AI is used more widely than others. In many cases, financial institutions are not far from using AI and the banks are actively investing in this area. There are a lot of FinTech companies as well which are built on AI.
The audience was very interested in what AI could do for them. Here, I think the main problem, is that many of users need good data in order to adopt AI. Data is crucial for AI – when the data is not available, or it’s not reliable, it’s very hard to apply. If you ask quantitative classification questions and grouping or clustering questions, then that’s something AI does very well. However, one of the questions from the audience was whether AI could help to determine whether it AI could help decide whether it’s in international interest or not to strike a certain trade agreement. That’s not a structured question, and I don’t think AI would be able to answer that.
DP: Thank you and I think it’s very important to understand structured data and the core methodology behind how AI can help improve certain processes within trade. How is Tradeteq looking at utilising AI to help facilitate trading? Can you give me some very specific examples and the core benefits that we can see as a result of this?
MB: We focus our AI on the analysis of credit and payment risks in the supply chain; the investors who will help distribute these assets. They don’t have the systems available to analyse these exposures, which are very granular. Some of the ratios are quite small and they are short term. So, doing traditional human trait analysis for them is just not feasible. We use two layers of data on companies, which allows us to do credit analysis at the company level and also data resume by reuseable arrays of data, which allow us to predict the timeliness and the extent of repayment receivables. So, what our clients get is a scoring, which can give early warning signs that a certain trade relationship will be changing or that a certain receivable falls out of the old pattern, and that the expectation of payments need to be updated.
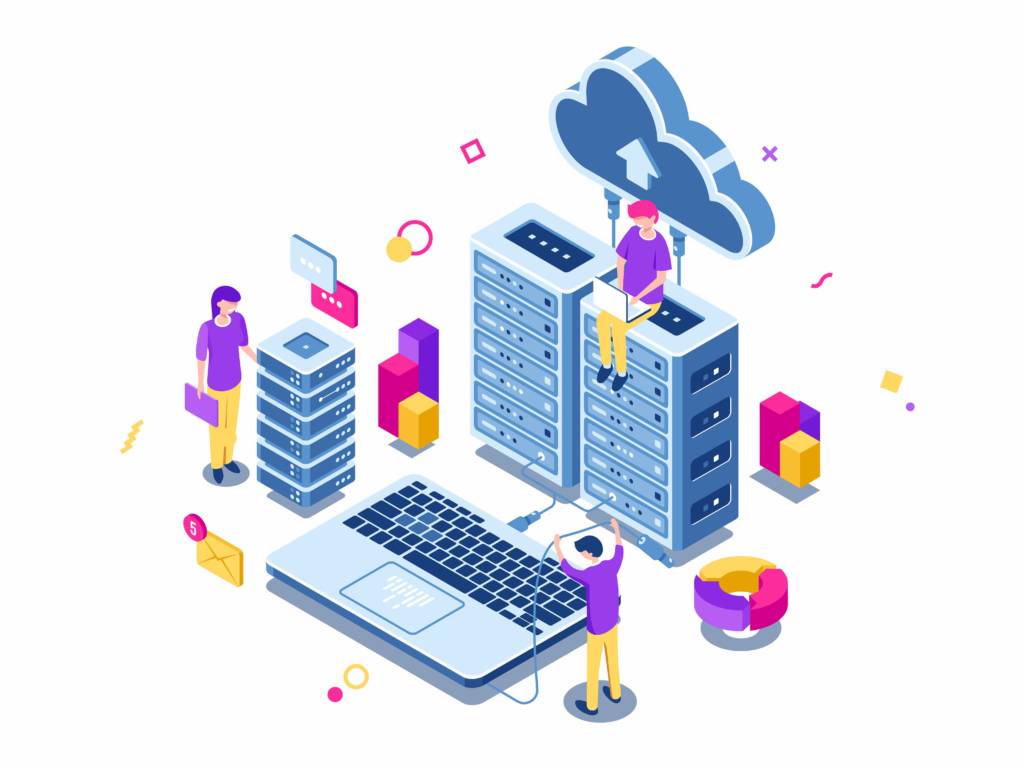
AI and Trade: The Challenges, and where machine learning steps in
DP: What are the top challenges with implementing AI within this space?
MB: The huge issue is missing data. Generally, when companies are in financial distress, they don’t submit data in a timely manner, in particular, they might not file their accounts on time. But assuming that if data is missing then it probably isn’t important is extremely dangerous. What we see in creditors in many cases, is that the pattern of how the data is missing is much more telling than the data per se. For example, when we look at small company accounts, we don’t really put that much faith in a single number on these accounts. Our models quite often pick up the presence or absence of a certain field and give it much higher importance than the actual value of the field if it is present.
So, in credit, generally this estimation of information is crucial and exploiting it correctly is important. The same applies to transactions. If an originator shares us with a data set, which just shows the positive experiences that they had with clients, we will be able to calibrate a model which predicts the likelihood of a negative experience. The model has to learn from the data. It is difficult to be unbiased but getting data sets with the controlled biases is important for us.
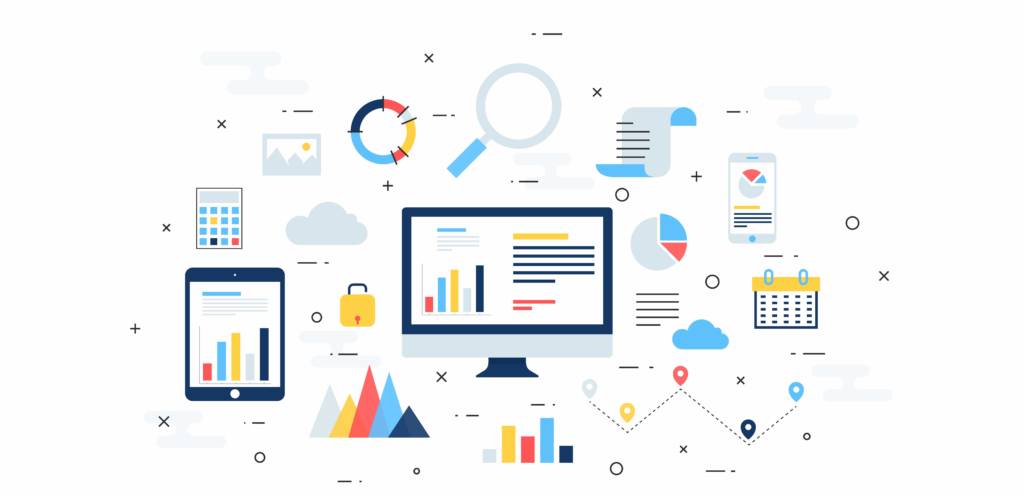
Industry Adoption of AI – Where are we at?
DP: Yes, I think the availability of clean data is potentially something that the industry lacks and it is very interesting that you guys will give the quality of data or missing data a particular score based on what type of company it is and where that data point is from. I still think that these qualities are a larger issue for AI and trade and receivables in that the systems aren’t connected, and finances and banks aren’t connected with each other. AI requires huge data sets, many of which we might not be able to access enough data to be statistically significant. How ready do you think our industry is to embrace AI right now?
MB: We have large digitisation initiatives at the financial institutions – which they are able to access in one place – their database of historical transactions. We have very important openness initiatives across governments and non-government organisations with an increasing number of countries, publishing the corporate data in the common format. We have the Internet of Things starting to facilitate the tracking of goods, which is quite important for assessment of trade finance risks.
We also have a number of initiatives in the blockchain space, which don’t have yet the data, but at some point they will be filled with the data in a uniform and accessible format. When people with the right to access privileges will be able to access this data in a relatively unified way, the rest of data will be improved, relative to the current state by the blockchain technology. It’s not there yet, but when it is we’ll be ready to mine the blockchain for data.
A summary of the key blockchain initiatives in the trade finance space
DP: Very interesting. I guess it’s a relief to know that you’re not mining for bitcoins, and AI and machine learning can offer a completely different solution within the trade and finance base to counter terrorist financing, and also anti-money laundering in a compliant way.
AI for Compliance and Regulation within trade / receivables finance
MB: Yes, actually, a lot of the analysis we do is very similar to the analysis that government and non-government relations do to uncover money laundering networks, which is look at the connections between companies. We’re not focusing on the anti-money laundering at the moment, but a lot of the analysis is similar – it’s just that we’re not searching for the transactions. But we focus on breaches due to all kinds of causes, and they can be perfectly compliant and from satisfactory companies, which nevertheless breach the obligations.
DP: So how long do you think it will take before the industry adopts AI as part of a mainstream offering in trading receive was financed and what exactly do we need to do to get to that point?
MB: I think the industry is getting there. Our clients are already using our AI tools to assess the risk of companies and transactions. Many other companies are doing the same, the originators, the banks, and non-banks are increasingly moving to AI driven systems, which at least assist the humans making the decisions. Within a year or two we will see a much more widespread adoption of these tools. There are a number of problems we need to resolve, and I think regulation will be increasingly important in this case. The issue of fairness will also be increasingly important, especially when AI is used for actual decision making and not just a visualisation or a system tool. We are looking at these issues and did a fairness audit analysis for our models recently. We are also looking to monitor that and take action when we see an unfairness manifested in the recommendations and decisions.
DP: Very interesting. I guess things like machine learning could be dangerous in the sense that they could create some form of bias or polarisation of decision making, which could actually end up prohibiting access to finance for certain organisations.
MB: Yes, it can be dangerous, but it can be also quite beneficial. The biases can be created, but also the biases are eliminated by machine learning. The machine learning has the advantage that one has to formulate the definition of fairness very clearly to even try to check the model for fairness, and once one defines it clearly, then one can look for resilient ways to combat that. For non-machine learning, there are people who generally disagree on the notion of fairness which prevents them from doing remedial action.
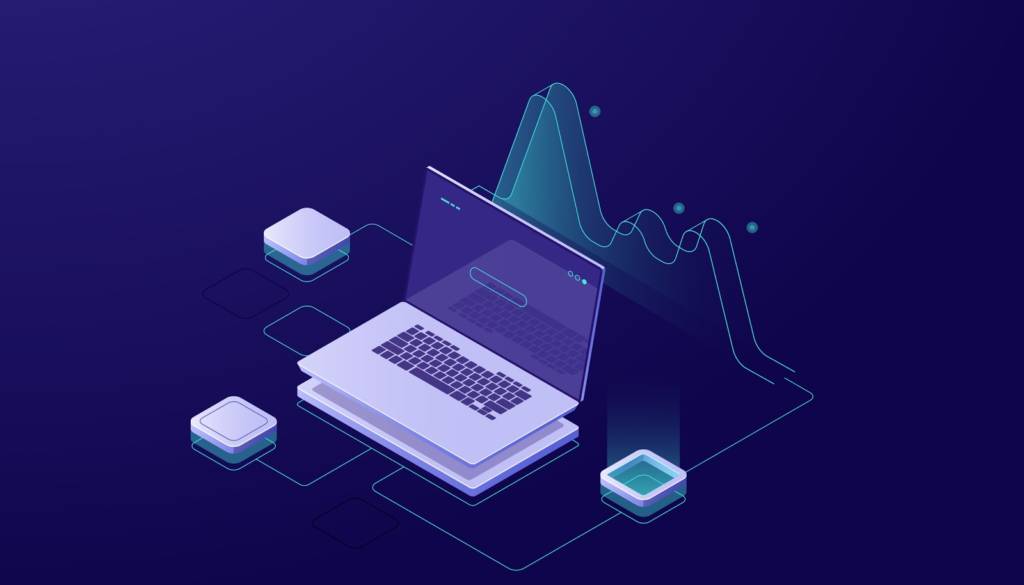
DP: Thank you very much, Michael. I’m afraid that’s all we have time for today, but it’s been amazing having you here and learning about how AI can be implemented within the trade and receivables finance sector. Understanding bias and which parts of the data contribute to decision making really needs to continue to drive AI within the space and make a positive change. So Michael, thank you again for coming and joining us today at the Telegraph’s Trade & Export Finance Conference. It’s been great to have you here and I’ll be catching up with you in the future to re-evaluate where we’re at in the industry! It’s been a pleasure talking to you.